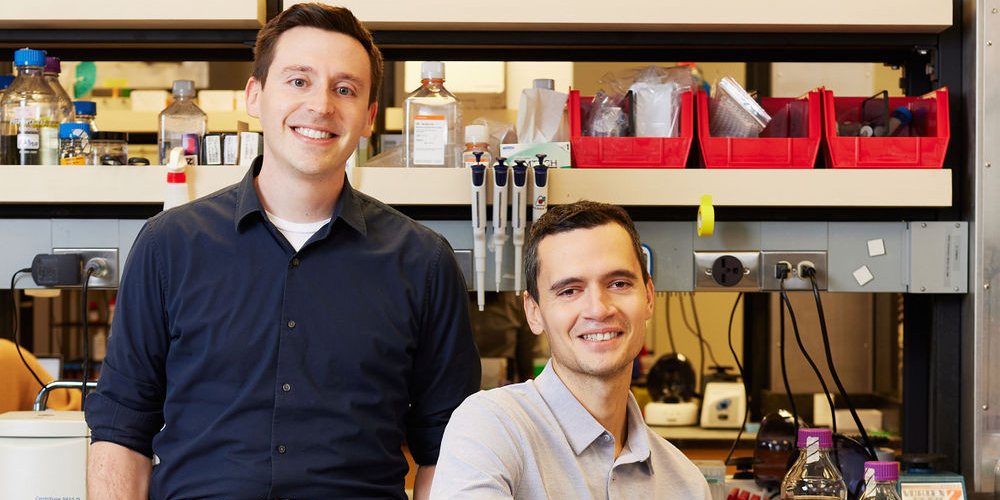
Over the last six years, Madrona has been investing in founders building companies at the intersections of life (biological and chemical) and data sciences – something we call the “Intersections of Innovation,” or “IoI” for short. Companies integrating the increasingly high throughput and complex life science datasets with the abundant and pervasive computational and data science resources are becoming a core part of Madrona’s investment focus. While we have written extensively about our “Intersections of Innovation” portfolio companies, it is time to share more about the overarching themes guiding our investments in this space.
Applied machine/deep learning and the life sciences are coming together to transform how the world understands and improves human life and health. We believe the intersections of these previously tangential fields will define breakthroughs in therapeutic research, diagnostics, clinical processes, preventions, and cures. And we are partnering with world-class teams focused on building wet/dry lab platforms and novel datasets to disrupt traditional drug discovery processes and mindsets while helping to solve the next generation of challenges in biology across areas such as proteomics, gene editing, synthetic biology, and more.
Why now
Madrona has been the vanguard of myriad technological paradigm shifts over the last 25+ years — from cloud computing to the explosion of open-source software to the emergence of intelligent applications. We believe we are on the cusp of such a shift in the life sciences. The status quo in life science is no longer enough. Today, drug discovery is a slow, painful process. Technologies to profile individual patients with unique “-omic signatures” can now deliver on the promise of personalized healthcare, but the data analysis and infrastructure to deliver personalized insights lags. On the therapeutic side, it can take $1-3 billion and more than 10 years to take a drug to market — and more than 90% of drug candidates fail along the way. That is not the way forward. Drug development can be accelerated through better target selection, low latency, biologically relevant screening, targeted clinical trials, and pharmacogenomics drug selection.
In recent years, biology, chemistry, and data modeling advancements have begun enabling teams to discover, validate, and advance science faster than ever before, but the sheer scale of data generated has become a bottleneck. Key innovations in lab automation, microfluidics, and translational models, for example, have enabled a much faster throughput for wet lab experimentation. Similarly, the technological revolutions in single-cell and spatial biology are generating complex datasets at an unprecedented rate These wet lab advancements create acute pain points that data science and computational approaches are uniquely qualified to solve: too much data, too fast. Only by applying machine learning to these biological data problems will the industry be able to leverage the appropriate speed and scale to realize the promise of personalized medicine, yielding a new standard of care — one designed for an individual.
What we’re excited about
We are beginning to see the impact of sophisticated machine learning models on crucial biological processes that are revolutionizing drug design, but the stage is set for so much more. With digitized data flowing faster than ever, innovative teams can use modern cloud computing resources to process, store, and analyze that data. They can then use this data to train a machine learning model to start predicting behavior — a protein molecule’s binding affinity to another molecule or structure-function relationship. That then enables more targeted wet lab experimentation that, in turn, improves the results and the whole discovery process. This creates an iterative loop that will improve both the traditionally manual wet lab process AND refine the ML model, accelerating drug discovery by many orders of magnitude.
As the fields of machine and deep learning improve, we can begin to see how the once brittle and simplistic computational models of biological systems become more sophisticated and biologically relevant. Running these processes at scale enables companies to generate novel datasets that rapidly improve prioritization of which potential candidates on biological targets of interest are worthwhile to advance to additional research or clinical trials.
For example, A-Alpha Bio, one of our portfolio companies, screens and analyzes hundreds of millions of protein interactions and then uses that data to computationally predict binding behavior. This process previously required scientists to screen two proteins in a single experiment and attempt to measure whether they bind to each other or not — possibly having to go through thousands of one-to-one experiments to find a match. A-Alpha’s ability to screen proteins at high throughput in silico (computationally) and in the wet lab has the potential to reduce the time, cost, and risks around the drug-discovery journey. This new, data-rich computational model paired with innovative wet lab screening can advance drug discovery in diverse fields ranging from antibodies to small molecule molecular glues. Computational models are not limited to drug discovery. Opportunities abound to deliver on the promise of next-generation human health in clinical trials, companion diagnostics, consumer health, and more.
Why the Pacific Northwest?
The next generation of intersections of innovation companies need talent that spans both the life science and computer/data science worlds. As the home of world-class technical talent in both areas, the Pacific Northwest is an extremely exciting place for entrepreneurs and a place we’re proud to call home.
Seattle is home to incredible talent in the life sciences ecosystem. The University of Washington hosts the world-renowned Institute for Protein Design (IPD), the Institute of Stem Cell and Regenerative Medicine, and the Molecular Information Systems Lab. Additionally, the Fred Hutchinson Cancer Center, a research and clinical facility recognized as one of the best in the country, has more than 100 labs under its roof and is home to multiple cutting-edge research efforts in machine learning.
Scientists driving innovation in their wet labs is not new, but the speed of discoveries that data science techniques introduce means that, as David Baker, who leads the IPD, articulated recently, “brilliant students … used to all want to leave the lab and become professors…now, they all want to start companies.” The combination of science, data science, and personal ambition to turn academic work into something that touches lives creates opportunities to build companies – providing researchers an opportunity to be at the forefront of both discovery and implementation.
On the computational side, the Seattle area is home to Amazon and Microsoft and tech hubs for Google, Meta, and many others. We’re in the cloud capital of the world, which produces no shortage of new companies applying machine learning in innovative ways and no shortage of tech talent. The Allen Institute for AI also attracts technologists and scientists looking to build companies, providing them access to researchers in artificial intelligence working at the top of their fields.
We’re seeing more technologists and scientists working across disciplines to be a part of an early movement of applying various engineering disciplines to the life sciences. We’re seeing software engineers increasingly drawn beyond the traditional tech industry and into the life sciences by way of the real opportunity to make impactful improvements in human lives and health outcomes.
Why Madrona?
Madrona has invested in ten intersections of innovation companies since our first investment in 2016 in Accolade. Several years later, we invested in Nautilus Biotechnology and began actively leaning into the intersection of life and data sciences. Today, our portfolio companies like Nautilus, A-Alpha Bio, Ozette, Terray Therapeutics, Envisagenics, and others are paving the way for the discovery of new disease treatments through the intersections of these disciplines. But, in concert with the field, we are just getting started.
Madrona is in the unique position of living in both the tech and life science worlds. We’ve spent 25 years working with SaaS, cloud computing, and intelligent application companies. We understand machine learning and how it applies to cutting-edge science. Applying our experiences to help life sciences innovatively combine wet and dry lab experiences and transform how the world understands and improves human life.
There is enormous potential in this space, and we are excited to back teams applying machine learning to the life sciences in innovative ways that unlock novel insights and solve problems across the life science and healthcare spectrum. We are always looking for eager entrepreneurs who embrace innovative new ideas – if that is you, please reach out to Matt McIlwain, Chris Picardo, Joe Horsman.